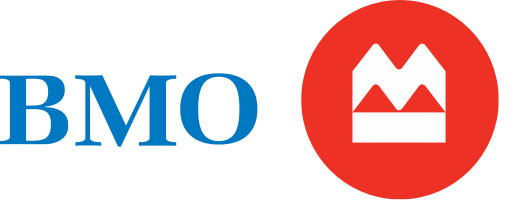
Your AI Roadmap: Who Else Should Be on the Journey?
-
bookmark
-
print
- Keywords:
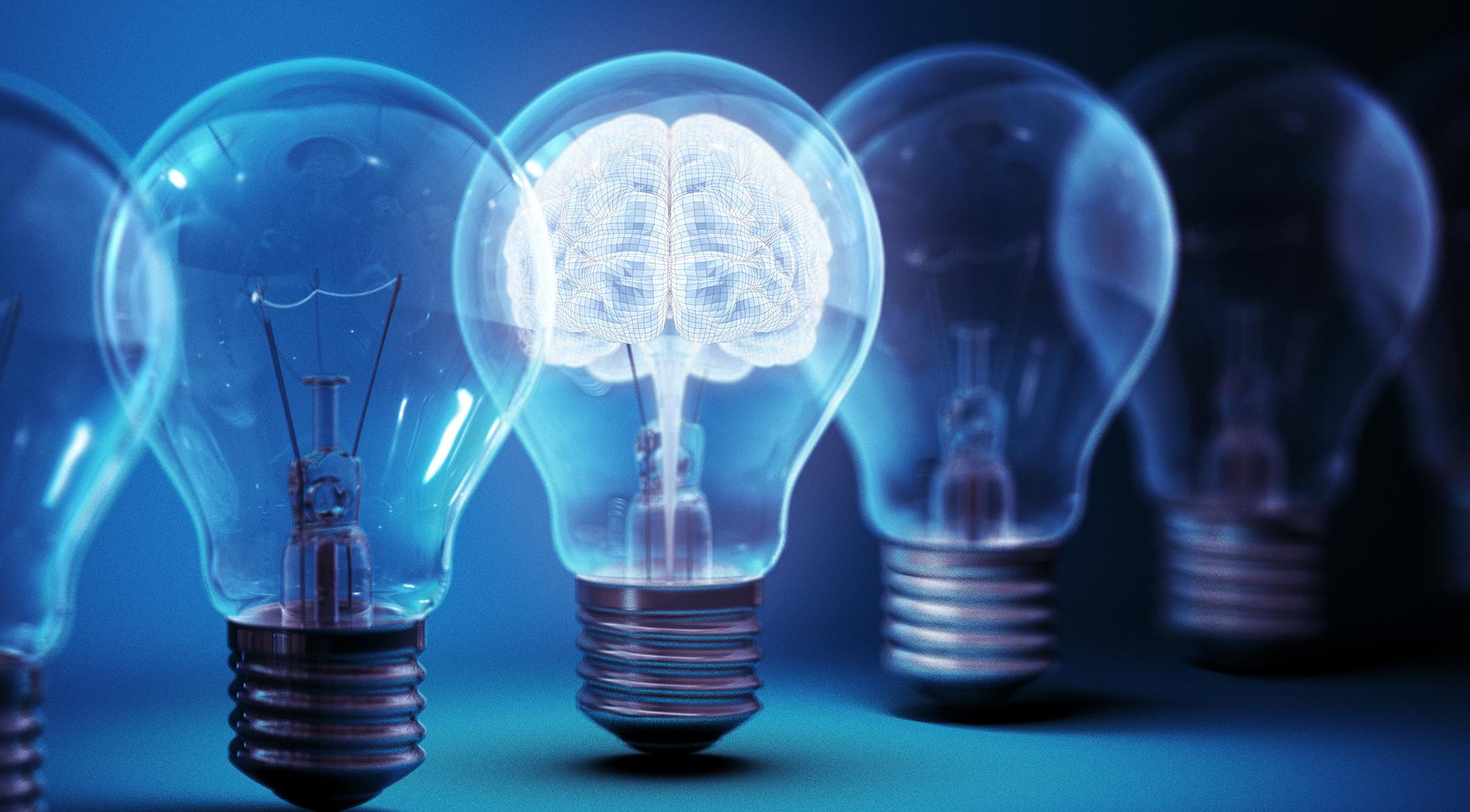
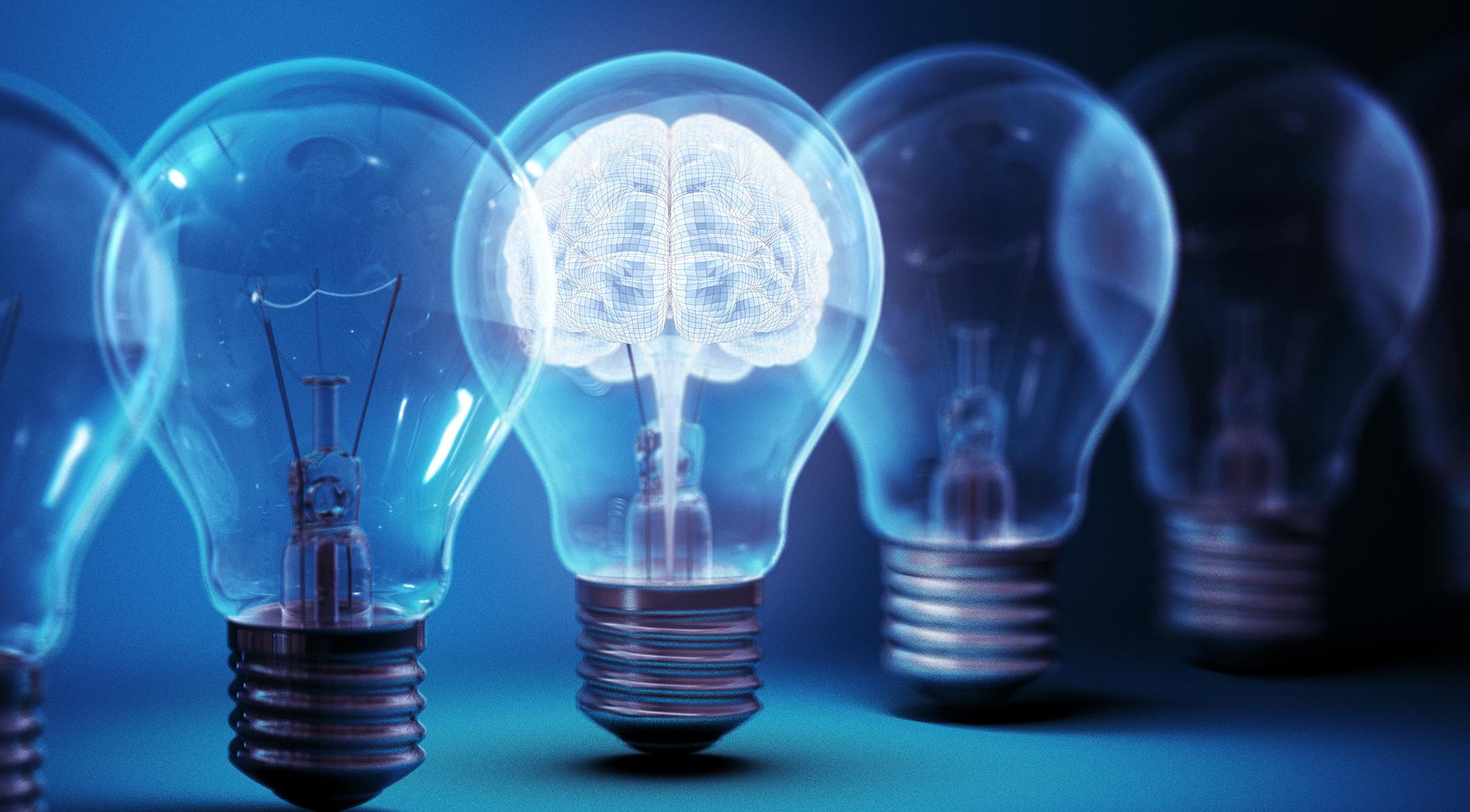
Contents:
Businesses of all stripes are exploring opportunities to use artificial intelligence (AI) to help solve complex customer problems. Most companies know that AI is important, but they’re not exactly sure how it could make their operations more efficient or how to keep their business safe.
To get beyond the noise, we hosted a webinar with a pair of BMO experts to discuss how companies can harness this emerging technology, including which stakeholders should be involved in the decision to integrate AI into your operations. Our panelists were:
- Paul O'Donovan, Head, Data Analytics and AI Risk Management, BMO
- Marc Pfeiffer, Head, Data Foundations, Innovation and AI, BMO
Sean Ellery, Head of Digital & Innovation Commercial Banking, BMO, moderated the discussion. Following is a podcast and summary of their insights.
Markets Plus is live on all major channels including Apple, and Spotify
.
Start listening to our library of award-winning podcasts.
AI’s business use cases
AI has been around for about 70 years, but advances in computational capabilities and the more recent focus on generative AI tools such as ChatGPT and Google Gemini have made it a part of mainstream conversation. And over the last several years we’ve all been encountering AI in one form or another in our daily lives, whether it’s the auto-correct feature on your mobile phone’s texting app, what-to-watch-next recommendations on streaming TV services, digital personal assistants, or smart home devices. These tools are largely designed for convenience.
From a business perspective, Pfeiffer said AI's potential benefits are largely focused on three areas: cutting costs, mitigating risk, and increasing revenue. To achieve these outcomes, he explained that various industries use AI to provide insights for making better decisions.
It helps you understand the patterns you’re seeing in the data more quickly, with more detail and with more nuance, and then getting those insights from management to customers in terms of spotting trends or making recommendations.
-- Marc Pfeiffer, Head, Data Foundations, Innovation and AI, BMO --
O’Donovan noted that while many companies are leveraging generative AI's automation capabilities to improve efficiency in certain tasks, such as document review, the next step in AI’s evolution will be the transition from a tactical tool to a strategic enabler. “The question that remains is that once these tools are in place and these efficiency gains start to get realized, how do you turn that into hard dollars and cents in terms of an impact to your bottom line,” O’Donovan said.
AI’s limitations
The potential benefits of AI are significant, but so are the possible pitfalls. Pfeiffer pointed out that responsible use starts with a foundation of quality data.
“If you have poor data, if it’s not captured properly, if it’s somehow biased, those are things that are going to lead to inaccurate predictions or faulty decisions,” Pfeiffer said. “One of the things we think a lot about with AI is explainability. You don’t necessarily need to know why the AI decided that a certain Netflix show was a good recommendation. But in other situations, it would be critical to understand why that recommendation or outcome was made. You have to be careful about which AI model you use; some have good explainability, some don’t. It’s something you need to think about, particularly in regulatory or internal compliance situations.”
O’Donovan noted that the risk of biased data is especially important to keep in mind.
These are complex systems that have a lot of black box elements to them. Understanding how your data is moving within the system, how you’re making sure it’s not treating customers or employees in different ways across different protected classes is critical.
-- Paul O'Donovan, Head, Data Analytics and AI Risk Management, BMO --
Upskilling treasury departments
That’s why as more companies adopt AI, some upskilling will be required within corporate treasury departments. Everyone won’t need to become computer scientists, but data literacy, critical thinking and ethics awareness will become essential skills.
As an example, O’Donovan said treasury teams will need the ability to spot where AI functions are not working as expected and know how to relay that information to the development team. “I do see some need for training folks within treasury departments to be able to appropriately interact with the AI system and be able to interpret and flag the output,” he said.
Pfeiffer also cautioned against overreliance on AI, particularly in situations that require nuanced communication, such as customer service.
If you have a chatbot communicating with a client, you need to be able to recognize when an AI isn’t able to handle a question and that it needs to go to a human who has a more nuanced understanding of the business
-- Marc Pfeiffer, Head, Data Foundations, Innovation and AI, BMO --
In its current state, AI functions well when it supports human efforts and work, so the lines of accountability are clear. “In certain cases where you're dealing with sensitive business processes or sensitive information, it’s critical that AI is seen as an enabler in the decision-making process and not the ultimate deciding factor,” O’Donovan said.
Who should be at the table?
As businesses look to leverage AI to support strategic needs, involving key stakeholders early in the process and communicating your goals are crucial to a successful implementation.
Many parts of the organization can be impacted. Ensuring that you have that buy-in early on is going to be a critical factor.
-- Paul O'Donovan, Head, Data Analytics and AI Risk Management, BMO --
To that end, O’Donovan listed the key groups that should be engaged in these discussions:
- Risk management
- Procurement
- Legal
- IT
- Risk and compliance
- Human resources
- Third-party technology providers
Along with getting the right stakeholders involved, cross-collaboration among these groups is also crucial. “What has really worked well is collective engagement,” O’Donovan said. “There’s a lot of overlap between the legal group and the third-party group, the technology group and the risk group. It’s important to have folks in the same room at the same time to make sure that all the different perspectives can be heard.”
Involving all your key stakeholders will help you navigate all the necessary considerations for a successful implementation. That includes making sure you’re using AI to solve for the right use case and that you’re using high-quality, structured data. It also means understanding how AI may impact your operations.
“The AI model is half the challenge,” Pfeiffer said. “The other half is making sure that what you're building will meet the needs of your business. Making sure you understand how it will be used, how the user—whether it's an employee or customer—is going to receive it, and how that's going to change their own daily operations as they interact with the company. Employee training and change management are critical pieces to making sure you understand the post-implementation functionality.”
Ultimately, knowing what you want AI to help you accomplish will drive all the other decisions that your key stakeholders will be involved in. “Companies tend to be less successful when there's not a clear set of criteria for success at the outset,” O’Donovan said. “Being clear on what constitutes a good outcome needs to be clear from the beginning so that all the different partners can be aligned on what the ultimate success looks like.”
JOHANNA SKOOG: Good afternoon, everyone. Welcome to the latest edition of NextGen Treasury. My name is Johanna Skoog and I'm the managing director and head of TPS I&CB with BMO in the USA.
For those of you who are new to our quarterly NextGen series, our aim is to provide great content and ideas on topics that are timely and relevant to treasury and finance professionals.
NextGen Treasury also provides the opportunity to receive CTP credits for attending each educational session.
To date, we've covered topics ranging from liquidity and cash management to economic outcomes and cyber fraud.
Today, my colleagues will be discussing another hot topic on everyone's mind: Artificial intelligence. Let's begin.
SEAN ELLERY: Thanks, Johanna. Thanks everyone for joining us today.
I'm Sean Ellery, head of digital innovation, BMO commercial banking.
My teams and I are always looking for opportunities to use open banking, AI and other emerging technologies to solve complex customer challenges, to drive BMO's digital first strategy forward.
There are almost 800 of us on this webinar today.
To me that demonstrates that we know AI's interest and importance, but we're not sure how it might be important to us specifically towards businesses.
After all, there's a lot of noise around AI.
Is it going to save the world or is it going to become self-aware, become the Terminator and destroy society?
Most of us understand that AI has enormous potential to benefit our companies, and we would like to understand how best to harness this technology, where to implement it, and where to see the most impact.
So that's what we're going to talk about today.
We'll start by defining what AI is, and what it isn't, we'll examine how AI is currently being used, and how to determine where it fits in your business.
Finally, we'll discuss how best to implement AI to ensure both short and long-term success.
We're also joined by two of my colleagues, both experts in this space. Marc Pfeiffer heads the data science and AI practice at BMO and commercial banking. He's responsible for the development and implementation of AI-driven solutions that optimize risk management strategies and drive financial growth.
Paul O'Donovan is head of data analytics and AI risk management at BMO. Paul leads the team responsible for enterprise risk management programs through data analytics.
Most recently, he led programs focusing on enterprise policy framework, emerging risks and complex transformation.
It's great to have you both here today. Let's start by defining our terms. Paul, we're talking about AI. What exactly are we talking about?
PAUL O'DONOVAN: Yeah, thanks, Sean, and AI even though it's only two letters really kind of covers a broad spectrum of advanced modeling techniques that people can use to gain insight and information from large quantities of data.
Really, in its simplest terms the way we think about it here is different ways in which data can be processed to help replace or inform human decision making and human inference.
AI by itself as a concept is not new. The term was first coined back in the '50s, but really what we've seen over the course of the past decade or so is a lot of advancement in technological capability, computational capability and a lot of focus particularly on generative AI recently in the last 12 to 18 months that's invigorating some of what you're hearing today in terms of the attention and focus on AI, and the different ways in which it can be helpful to drive growth and revenue opportunities across different types of businesses.
So as we think about AI, we really think of it across a broad spectrum. I think equally important is what -- what AI isn't, and I think how we scope that out from what we do.
AI you know, as I mentioned is really a broad sed of modeling capabilities, stopping short of more standardized normal technology capabilities and specifically things like robotics and process automation, which is typically outside of the umbrella of what we think about, and we really focus on the processing of large amounts of data to help inform inference and decision making for folks within our business.
SEAN ELLERY: So also for you Paul, what are the different types of AI? And what capabilities do they have?
PAUL O'DONOVAN: So I think again there's a spectrum here, and on the lower end of that, on the kind of less complex end and more traditional modeling capabilities, we really see some of those traditional machine learning methods. Things that have been around for a long time, used to do things like predict fraud, identify credit worthiness or assign credit scores to different customers. Some of your typical usages of classical modeling capabilities that have been around banks and other businesses for a long time.
More recently, I think some of the more complicated and involved applications that we've seen, such as deep learning and things like neural networks really are kind of focusing on trying to find hidden and complicated patterns within the data itself, and to draw more insightful information and predictions from these types of models.
And so we've seen a little bit of a pivot from traditional machine learning to kind of deep learning and different, more complicated algorithms.
I think more recently, though, a lot of the focus has been on generative AI, and particularly on some of the capabilities we see around natural language processing, some predictions, and generation of content, specifically be that through text, through image, through video, and really seeing that kind of permeate in a lot of different places.
You know, internally here and as we focus on things, we're seeing a lot of these use cases kind of come through from the perspective of trying to improve efficiency and make our daily lives a little bit better, and more efficient in how we work as a business.
SEAN ELLERY: Thanks, Paul.
Marc, let's hear from you. How are most of the encountering AI now in our daily lives?
MARC PFEIFFER: It's quite amazing how much AI has proliferated in our daily lives, personal assistants like Siri and Google Assistant types of things. Netflix, your recommendation systems that you'll see there, Amazon where you shop. Even credit card companies use it for fraud detection. You used to have to call into the company and say hey, I'm traveling to such and such a place for these dates and that's gone now. The AI will actually help to detect when you are abroad, and they don't need that anymore.
Social media, autocorrect on your phone. It's really proliferating pretty much everywhere in our daily lives.
SEAN ELLERY: Let's shift to a business perspective.
How are different industries using AI to optimize processes or solve challenges? How are they putting it to work for them?
I'll start with Marc.
MARC PFEIFFER: Thanks, Sean.
Maybe I'll start by saying, you know, AI as a tool is while we hear about it a lot, companies haven't integrated it to great effect. Only 5% in a February poll of American companies had actually implemented AI. And that varies my industry, of course. Technology companies in the 20ish type Pennsylvania, manufacturing down 3%. You will see a lot of variability and it tends to be focused in the larger companies as of today, which is changing as AI as a service becomes more amenable.
But the way these companies are using it, I'll broadly put them into two buckets. Providing actual insights is the first one, helping to make better decisions, understand those patterns that you're seeing in the data, more quicker, more detailed, more nuanced, and getting those insights out to management or in the hands of customers in terms of recommendation systems, and things of that nature, help spot trends, that sort of thing.
The other area that you see this happening a lot is enabling key and differentiating functions.
So as you think about companies that typically operate like a manufacturing type of setup, they're using that to actually help redefine some of those core business functions, to create competitive advantage. Can they be logistically more efficient? Just in time of entry coming in, that sort of thing that really helps them create some advantage both financially and operationally be more efficient.
And so, we're seeing that a lot, customer service I would say, chat bots, we've seen that kind of change in how we're serving our customers, from a supply chain management, as I mentioned optimizing those logistics, enabling more efficient inventory management, delivering faster, better cost control, that sort of thing.
So those are probably the two broad areas that I see it in.
SEAN ELLERY: Okay, Paul, do you want to add any additional comments?
PAUL O'DONOVAN: Sure, I would say maybe in the more recent kind of applications of generative AI specifically, in addition to what Marc just said, really where we're seeing that at the moment is more kind of on the productivity and efficiency lens of things.
So, can we get more efficient with automation and deploying generative AI to replace kind of mundane repetitive tasks across different types of functions, particularly support functions that rely a lot on things like document review and consolidation.
And so really, we're seeing a lot of the use of generative AI in that space at the moment.
I think the key question that is still kind of out there in terms of the ultimate utility and value realization from a generative AI perspective is once these tools are in place and these efficiency gains start to get realized, how do you actually turn that into kind of hard dollars and cents in terms of an impact to your bottom line?
And so there's a lot of investment, a lot of I think investigation and proofing out of these different types of tools, and I think we'll start to see kind of some of that morph into -- once those efficiencies and productivity gains are there, how they're realized will be the next way that we start to see some of that take shape.
SEAN ELLERY: Thanks for framing that.
For both of you and I'll start with Marc and there's a lot of possibilities clearly. What about limitations of AI?
Are there areas where we should not use it or at least use it with a few caveats or guardrails?
MARC PFEIFFER: Yeah. I mean, there's a few things that I would mention here. The first, the foundation for pretty much any AI is good data. So having good-quality data, having it available to the data scientist or whoever is going to be building these models is critical.
If you have poor data, it's not captured somehow, it's biased, those are going to lead to faulty decision making.
There's ways you can control for that.
Having programs in your company that think about data, making sure that you regularly monitor it, you've got problems to make sure that it's validated, and essentially, that it's good quality.
One of the other things about AI is we think about a lot is explainability. In certain use cases, you don't necessarily need to know why the AI decided that a certain Netflix show might have been a good recommendation or not. But in other situations, it would be really critical to understand why that recommendation was being made or why that outcome was being made.
So you have to be careful in which AI model you use. Some have good explainability, some don't. And you don't want to end up building something in a black box, and then needing to have an explainability there.
Particularly in regulatory types of situations, or other sensitive internal compliance type of things, that is a critical part, and certainly, for us here at the bank, we think about that a lot.
Another area that we have to be careful on is overreliance on AI. It's good and it makes good predictions, but it's not always right. And certainly, in nuanced cases, like if you're dealing with a chat bot type of situation with a client, in very nuanced situations, you need to be able to recognize when you aren't actually able to handle that question right and it needs to go to a human who has a more nuanced understanding of that business and can really understand the factors involved there.
And so building that cross-over, and understanding how you're going to handle, call it bad predictions or bad outputs, is something we need to be mindful of.
And the last point I would make is around cost. It can be expensive, depending on how you're approaching it, what you're building, etc.
That ROI point that was brought up earlier is really important, and so I don't want to say you have to throw AI everything, because certainly, from a cost point of view, that won't always be tenable.
PAUL O'DONOVAN: And I think from my perspective, just to add a couple of points to what Marc has already mentioned. I think this point around not replacing human judgment is really, really important, and so in certain cases, that may be kind of very clear in terms of where it can be used to automate some decisioning and interact directly with consumers of the output of that AI system.
In certain cases where you've got sensitive business processes, dealing with sensitive information, you have critical decisions to make, it is really critical that AI is seen as an enabler in the decision-making process and not the ultimate deciding factor at this point.
I think all of the things that Marc mentioned around data explainability, overreliance, are really important to highlight this concept of maintaining a human in the loop, or having a person interact with that decision making when it makes sense to do so, when you've got certain types of processes in place.
I think that's a really, really critical point.
Ethics and biases is one that's really topical right now within our industry, and I think across others, this concept of ethical use or responsible use of AI I think is really, really important.
These are really complex systems that have a lot of kind of black box elements to them and so understanding how your data is moving in, how it's moving within the system, how you're making sure that it's not treating customers or employees in different ways, across different protected classes is really, really critical.
So again, the ways in which it's being used is really important here.
And then, the final point I would make is just from a regulatory and compliance, and Marc touched on this a little bit, but we're seeing more and more evolve globally and specifically within the U.S. even at the state level at the moment, in terms of the regulatory and legislative expectations around how companies can safely deploy AI and the protections that need to be in place for consumers.
And so the regulatory and compliance angle here is very, very important. It's a space that's evolving really, really rapidly.
The EU AI Act was the first major landmark law that came through, but we're seeing more from a north American perspective as well so that will be a key space to focus on in terms of again the ethical and appropriate use case of AI, but also where prohibitions can be in place from a legislative perspective.
SEAN ELLERY: Those are really great points to consider. Thanks for sharing them.
With all of that in mind, what's the best way to determine how AI might benefit the business?
I'll hand it over to Marc to start.
MARC PFEIFFER: Thanks, Sean. I think most of the time we approach this almost like any other initiative. As we think about technology or building a process or outsourcing or any of those other types of things that we consider, AI is actually not that different at the end of the day. We look typically to see where we can save some costs, what's our biggest cost driver? Is there a possibility of applying AI to that? Where is there risk in our business? Or where are there revenue opportunities? I see some models where specifically customer experience and employee experience are called out as differentiating factors to consider.
Once you look through those types of dimensions, you can start to home in on the areas of your business where you're ultimately bearing those costs, or you've got some potential to grow.
And that's where we really focus on.
And so I would call that the demand side, where we think about where is there a need for it?
And then, on the more supply side, I'll call it the build. Whoever to get there?
And back to the ROI point that we mentioned earlier, you need to prioritize based on that complexity of implementation, the costs of that implementation, what does it mean? How disruptive is it going to be to your business, to your employees, to your clients, versus the benefit that you're going to get out of it?
And there's a fine line there I think where you can overindex a little bit on AI, and you really need to make sure that implementation is going to be how you envision and the cost and the risk that you're comfortable with.
And part of that really is involving your stakeholders early and getting that buy-in and making sure folks understand what you're trying to do, why you're trying to do it.
As we talked about the different areas that this can impact, when we think about human resources impact, technology impact, legal impact, risk impact; there's really a lot of parts of the organization that can get impacted here.
So ensuring that you have that buy-in early on and understand how your build of AI is going to implement and integrate that is going to be a critical factor.
SEAN ELLERY: Paul, what do you think?
PAUL O'DONOVAN: I think those are all really good points. I think I would probably add, too.
As we look at AI, and I think in general, as different businesses are looking at it, it's important to consider that AI can be an effective enabler of your strategy; it is not by itself the outcome to strive for.
I think that's the point that Marc was making as well, where, you know, it's really about finding the right use cases in the right ways that the technology can be leveraged to improve the efficiency of your business or kind of drive the demand side as Marc was mentioning.
I think embedding it within the broader business strategy in terms of how you're advancing, how you're adopting technology, how that fits within the capability of the business as it is today or kind of will be in the future, and I think critically as well as the appetite in kind of the responsiveness of your employees and customers for what they're actually looking for from a service perspective, as well.
I think that's really important for considering how AI can be leveraged across different businesses.
And I think really on that point again, of, you know, making sure that it's an enabler of strategy, not in and of itself the strategy, because oftentimes, I think there's a focus on AI as the solution to every problem, when the reality is very different.
And oftentimes, simpler, lower cost, smaller scale technology builds, or chat bots are a good example of this where you don't necessarily need a large-language model with a chat bot to interact with employees or customers and oftentimes, there's a much simpler, more cost-effective solution that can get you 80% of the way there.
So I think really kind of stacking it up against other options and making sure that it's an enabler and not an outcome by itself is really important.
SEAN ELLERY: That's really helpful. As we think through our discussions we've had, the next question is pretty near and dear to my heart and I know we've had a lot of conversations here within BMO as we think about sort of the next steps in establishing road maps.
Once we've established where AI is most impactful for your business, you need to create a road map to know where you're headed and how you're going to get there.
Paul, I'll hand it to you, because I know we've had a lot of conversation on this particular topic.
PAUL O'DONOVAN: And you probably don't like the answer that it's going to be a lot of people that need to be engaged in this process, but I think given the newness of the technology, that's to be expected.
I mean, some of the key groups we've been engaging from a risk management perspective as well as we look at this and making sure they're part of the process, procurement and third party groups are going to be really, really critical to this. The reality is, given the scope and scale of some of these solutions and what's needed, third-party engagement and support is going to be inevitable as firms look to embed AI. Whether that's in the procuring of cloud-based services or whether that's in the actual onboarding of specific solutions of your members, but the procurement group is going to be very critical so making sure they're engaging in the process is really important.
The legal department is also going to be critical here. I think one of the things that we come across a lot is the concept around the intellectual property for the business, making sure that that's protected throughout the life cycle of these solutions, maintaining kind of appropriate protections within contract language is, obviously, very important as well as you're engaging with different vendors. And so the legal group is going to be very important as part of those discussions.
You know, probably one of the biggest ones is technology, Marc touched on this already from a supply side perspective, but technology capabilities and particularly how AI can be deployed within your existing stack is going to be very, very important.
That matters from just a technology perspective, from a cyber security perspective, an information security perspective. So CTOs, CIOs, CEOs are going to be very important players in how you're thinking about executing on this.
And then, selfishly I'll have to call out the risk and compliance functions, because those are, obviously, very important partners here, as well as the road map is being developed.
I think from a compliance perspective again we've touched on this. This is a very hot topic globally with a lot of regulators and a lot of legislators so being mindful of what those changes are is going to be very, very important.
From a risk perspective, understanding the right controls around how this is going to be deployed, how information is going to be protected, how we're going to ensure ongoing efficacy of some of these solutions as they go in.
I think a lot of these AI solutions move to scale and move to deployment, there has to be some process around monitoring and making sure that they're continuing to perform, and not having a "set it and forget it" type of approach to deployment.
And so those are some of the key groups. I think there will be other stakeholders, potentially HR departments and different folks that need to get engaged, depending on the type of use case that you're talking about and the type of deployment that you're talking about.
Those are some of the key ones that we've worked with.
And I think in our experience, what has worked really well is the collective engagement, and so having a forum where these folks come together to speak through these solutions collectively is very, very important.
There's a lot of overlap between, for example, the legal group and the procurement third-party group, the technology group and the risk group, and so having folks in the same room at the same time is very important as well to make sure that all the different perspectives can be heard through the discussion of some of these solutions and the road map.
SEAN ELLERY: Yeah, absolutely.
And Marc, what about achieving the broader value?
MARC PFEIFFER: Yeah, it's a good point, and to pick up on where Paul was just going, although you need to get all those parties to the table, you don't want to do that repeatedly within the organization. So how do you think this as an enterprise-wide capability? Like invariably any company will start building it, somebody is going to start and want to grow that, and I think that's okay. That's a fine way to start.
But you really have to start thinking about how are we going to have a unified technical infrastructure, so that you can scale this? And not just the technical piece, but to Paul's point around all those parties at the table who have to opine and put their perspective into this to create a great product, it's going to fit within the organization.
You really want to start scaling that across the organization, as soon as you start to get there. All the technology, as one department solves a problem or develops a unique approach to something, that can be leveraged, or just directly built upon as well, great things to do.
You do want to build once and apply many times and as Paul mentioned, having that governance to kind of tie it all together, make sure that we're all operating ethically, applying all of our rules and establishing that governance framework I think is really critical.
And those are all enterprise capabilities. So you start small, but then quickly think about these things as foundational to how you're going to scale that across the organization.
SEAN ELLERY: That's great. Keeping with you, Marc, once you receive buy K-9 from your key stakeholders and it seems like there's a lot of folks to make sure they're at the table in providing their insights and their expertise, what are some of the key considerations you need to be aware of around an implementation?
Especially if you're a small or midsized company. Are there any major pitfalls you want to avoid? Marc, if you can provide context and Paul if you want to add.
MARC PFEIFFER: Sure. So picking the right use case is probably the first thing that we really, as an organization, need to home in on.
I think it's important to start small, learn and grow. As we've discovered internally on our own AI goals here, what we think of as the best thing or best outcome for a model is really geared towards the data that's available, what's it telling us, what are the types of insights that we can get out of there?
And so starting small, learning and growing I think is critical piece to the equation to start off with.
You want to as I mentioned have really high-quality structured data. That's going to be key to emulate AI implementation. If you just don't collect the data today, or you don't know that it's of good quality, that's just going to be a nonstarter. So you need to make sure that you have those building blocks in place, you know what you want to build, you've got the data infrastructure to build it, and then, you can start building those things.
The other area that's really critical here finding the AI model is half the challenge. The other half is the successful implementation of that. How do you make sure that what you're building will actually meet the needs of your business? I've seen this in other places where like, for instance, a marketing model is built, and that then lands in the hands of the salespeople and they're looking then to work with it, and it just doesn't resonate, and they've had to go back then and re-create the models with those partners at the table.
And so making sure you're really thinking about the end-to-end, obviously, all the areas that we talked about, leading up to this conversation, but just making sure that you understand how it would be used, how the user, whether it's an employee or customer, will receive it, and how that's going to change their own daily operations as they interact with the company, how that's going to work.
So employee training and change management, I think those are really, really critical pieces to making sure you understand the post-implementation functionality.
PAUL O'DONOVAN: And I think Marc to build on what you were just saying, I think it's really important, one of the key pitfalls we see through some of the early engagement and proofs of concept that we run through, where things tend to struggle or tend to be less successful is where there's not a clear set of criteria for success at the outset.
And so being clear on what good looks like from the implementation and what you're trying to achieve with the deployment of the AI is very, very important, and very clear at the outset.
So business stakeholders, technical developers, technology, all the different partners in this can be aligned on what the ultimate outcome and success looks like from that solution.
The second thing I would mention, just in terms of pitfalls, I think one that I can't emphasize enough is really about protecting information. Obviously, companies have a lot of different controls, frameworks, risk management processes in place to make sure that their information or customer information, their confidential information that they use internally is highly protected, and with the deployment of AI and the complexity of how this works in terms of having to move data to the cloud and kind of leverage public technology to execute a lot of this stuff, doubling down on that information protection is going to be very, very critical.
And so again, the pitfalls to look out for there really are around understanding as you're deploying this AI and as you're leveraging cloud-based or third-party based solutions, how your data is moving, who has access to that and at what point, what protections are you going to put in place to make sure that there's not any unintended use of that data or any unintended use of that data.
This is another area where we're seeing a lot of regulatory development more at the state level at the moment, but definitely through EU, and some of the pending regulatory and legislative changes in North America, a lot of focus on privacy and information privacy and protecting customers' information.
And, you know, with the deployment of AI, that becomes more and more challenging, and so one of the key things to look out for for sure.
SEAN ELLERY: Thanks, guys.
Let's pivot a little bit to the future, because we all know technology evolves pretty quickly. Where do we see AI heading? And how do we want to make sure we don't miss out on it? Marc let me go to you first and then maybe Paul to add.
MARC PFEIFFER: Sure. So I think the places where I see it really growing, better predictive analytics, we talked about getting insights out of the data earlier today.
And I think that's just going to become better. The techniques and the algorithms that underlie this technology will just get better at predicting outcomes using probably increasingly less and less data and just creatively putting that data together, without getting to the specifics of it.
Natural language processing, I see that as another big area that will get better.
More understanding of the nuances. So when you read a sentence, understanding the context, of that, there's going to be some variability that's not captured today. I think that will get better over time.
And so we'll see tools like ChatGPT or any of the other kind of large language models, Microsoft Copilot, the full gamut that's out there, will start to get better at understanding what you're asking of it, what it's receiving, so you'll start to get better quality data. We'll start to limit hallucinations, that's a big topic today I would say in Gen AI where can you really trust that output? Because 80% of the time it's good, and the 20% or 10%, whatever it is, depending on what you're asking of it, where it gets it wrong, it sounds convincing, and so you have to really be careful about that, and I think that's the part that will start to sort of squeeze out as the technology matures a little bit.
We're also seeing more and more modalities, by modalities I mean text versus voice, images, videos. Certainly, the first foray into Gen AI has been text and we've seen a lot of images come out of that now. Voice is certainly a piece that will start to become more prevalent. You can chat real time, like us.
And we'll start to see that and that will have great application in places like marketing, where videos and advertisements will just be sort of generated on the fly that's very personalized and things like that.
And lastly from capabilities perspective, I would just say we're seeing more and more around an AI assistant where an AI is really augmenting you, as an individual, and we'll start to see more of that as we build in these platforms that are able to consume the data that we generate in our daily lives, and then, be able to use that to help us actually operate in our daily lives.
And that will eventually lead to the more hype side of things now, but I'm sure it will become real over time, agentic AI, which is really around that autonomy of an agent that can operate within your company to perform some tasks and so today, we're very much seeing assistant type focused things and that will start to move towards being a little bit more autonomous and certainly, start with low-level type of applications and then grow I think over time.
How well we get there and the controls that need to be in place, we'll have to deal with that when we come to it, but that's what I see where the industry is pushing the boundaries there.
From an application use case perspective, practically as a company, it will continue to optimize operations. Especially where anything is costly to run today, or the risk element to there, your manufacturing type of situations, it's going to help predict cart breakdowns, where you might need to be more proactive in maintaining the equipment and things like that. You'll start to see more of that come into play.
Personalization and customization for both customers and employees, we're already starting to see a lot of that as you log into your Netflix and Amazon, wherever else. There's more personalized recommendations coming through and those are base use cases that we have today, but we'll start to see more and more of that.
As you interact with any company, they'll start to get a perspective of who you are, what you can bring to the table or how you interact with them and be able to personalize that interaction a lot more.
And then, lastly, around intelligent decision support. So we talked about better predictive analytics and so we'll see that actually come to bear in the market. From a treasury context, I would see things like cash flow forecasting becoming very prevalent, understanding really what you're going to need from a cash perspective, manage of liquidity really well, and then, doing with that capital, the excess capital that you have and how you should deploy that optimally.
And so I think those are all areas that we'll start to see more and more this come in.
And then, to help enable that, we're going to see a lot more AI as a service.
So if you think about today a lot of the stuff gets built in house. There's some building blocks in the cloud, but more and more companies are starting to create bespoke solutions to solve a cash flow forecasting or parts breakdown type of prediction algorithm in the manufacturing context.
And those are all things that we'll start to see companies build as capabilities and start to offer as a service.
And I think we'll start to see those technology companies build up and then start to deploy in the market, because it often makes sense to do that, because then one company can tackle the problem, and then customers benefit from that.
PAUL O'DONOVAN: And Marc, all those are great points. And I think, from my perspective, kind of two quick points I think I would make.
I think in the short term, we'll see a little bit of convergence on some of the industry-provided tools. And I think when Open AI brought out ChatGPT, there was a big rush for some of the major technology companies to bring out their own version, and I think we'll start to see convergence on some of those tools and capabilities and we'll start to see some real winners emerge for particular use cases.
And so I think that will ultimately introduce a little bit of standardization across the industry in terms of what tools work best for what problems.
And so I think in the short term, we'll see that.
And obviously, kind of everything that Marc just mentioned about some of the advancements for sure. I think in terms of not missing out or kind of how best to engage on this at the moment, I think one of the key points here, and Marc touched on this around the hype is there has a lot of hype over the last 12 to 18 months, and I think those who will be successful at doing this will find ways to separate the hype cycle from true ROI and added efficiency gains.
So it goes back to the point we're making about AI in and of itself not being a strategy but being an enabler of your strategy.
So I think firms that can home in on opportunities that drive ROI as opposed to just drive AI technology out there into the world will really kind of be the ones that are kind of best positioned to move things forward.
SEAN ELLERY: Right on, thanks, gentlemen. We've seen some questions coming through the chat. Kind of around how do you evolve and keep pace with those changes? And specifically around the skills that are going to be important moving forward?
The question is should I have some of my folks in the treasury department learning how to code? Or is it just better knowledge of how things are evolving in the industry?
I'll pass it over to you Paul and Marc to provide some additional thoughts.
PAUL O'DONOVAN: Yeah, thanks, Sean. I think from a skills perspective, I think as firms get further along in their AI journey, there's definitely going to need to be some up-skilling across the business, in terms of really how people are interacting with the AI, how they can% use it to help their day-to-day operations and really I think more so also in the context of where to spot when things are not working as expected and how to funnel that back to the key development folks.
So I do see some need for training and up-skilling for folks within treasury departments and others to be able to appropriately interact with the AI system and be able to interpret and flag the output from that.
I would stop short of saying it translates to everybody needing to be an expert in AI or the AI system. I think ideally folks won't need to be kind of deep within the code or have specific knowledge about how things are working from a technology perspective.
I think centralized functions within technology, within some of the key AI groups, will continue to provide that from an enterprise point of view, and really within the businesses on the front lines, really being more versed in how to use the technology and how to provide feedback when it's not working as expected.
So again, I think some up-skilling, some proficiency, some technology proficiency is going to be needed across-the-board, but I wouldn't say it extends to the need to turn everybody into a computer scientist.
SEAN ELLERY: Marc?
MARC PFEIFFER: I think those are all great points. I would home in a little bit. Think about this at the employee level. The types of things that you would want them to be familiar with, data literacy, understanding the value of data, how it flows within the organization.
And to Paul's point, they don't need to be an expert on this, but it's the kind of thing that will help promote that good data culture at an employee level.
AI tool proficiency, depending on which AI you're thinking about deploying, if it's a large language model, like understanding its output and can you rely on that output? Or how do you handle those cases where it may not produce kind of the right output? And how do you work with that?
How do you train people basically to use a chat bot, an LLM as an example?
And then, ethics and -- (inaudible) are going to be critical from a control perspective as we think about certainly, the banking area we think a lot about. If we're going to put a model in, how do we make sure they're not biasing it against things that we should be biasing it against like race, gender, that type of thing so having that awareness of what that regulatory landscape, what our own internal company policy is will be really important.
So those elements, at an employee level, really important to get set up, and make sure everyone is kind of attuned to those.
And then, at a more company level, I talked about data a lot. I think that's absolutely a critical part. It's a part that probably in any implementation that we do we spend a significant amount of time talking about that data component, because it's really the foundation to AI.
And the AI is almost I don't want to say easy, but it becomes much easier to work with if you have good, solid data.
I think it's important to have it as part of the discourse, generally speaking.
As Paul said earlier, it's not going to be the be all end all within a company. And I spend in my position a lot of time talking about it, I recognize there's a lot of other parts of the organization. This is just one tool in the toolkit but it's an important tool, and it's important that we actually have that as part of regular discourse.
We understand what competitors are doing in this space and what we can do, but it's not going to be the answer to everything. But having that as part of that conversation I think is really important.
One lesson I've learned internally is that agility is really important. You really want to test, learn and adapt. If you have a sort of development cycle that you conceive of it, you write down the requirements, you build it and you deploy it, that tends to work less well with AI. You really need to iterate, practice, learn, adapt, and then scale, and that's going to be a continuous cycle that we'll have to do.
So that culture around agility I think is a really important piece to get there, and as you think about your own companies, how are you agile enough to take this on? And then start to experiment and change, because what you thought today might not be the answer tomorrow, especially with the fast-paced movement in the AI space specifically. I think that will be an area of agility and continuous learning, is just so important.
And then, lastly, third-party integration. AI as a service will be more and more prevalent out there, and that will unlock potential. So being open to that and understanding how you're going to interact with those third parties and the policies and data protections and so forth. These are all things that you can get ready in your organizations today to be best positioned to take advantage of AI.
SEAN ELLERY: Those are great points. Let's transition to now the wrap-up phase, and I would like to ask each of you to give us your top takeaways from the session, the key things we should take back to our teams. Paul, let's start with you and Marc I'll let you have the final word.
PAUL O'DONOVAN: Good to give Marc the final word.
I think I would say kind of three key things from my perspective. And we touched on each of these throughout the questions here, but really the first being treating AI as an enabler and not a strategy in and of itself I think is very, very important.
And so again, recognizing that it will help in terms of business process and efficiency and change kind of how we work, but it's not by itself going to be the solution to every problem and so I think recognizing that and building a road map around it as opposed to with that as a primary objective is going to be very, very important.
The second point I would make, you know, again, kind of back to the information and protection and data is really around as you're deploying AI, as businesses are looking at really focusing on information protection and making sure that customer employee and sensitive company information are treated very, very carefully, as it's interacting with these different AI solutions.
Again, recognizing that the underlying machinery and the complexity of the algorithms make it difficult to understand exactly how data is being transferred and moved throughout the system, and where it may be picked up.
It's still important to try to build the right controls and right risk management protocols around making sure that information is protected.
And then the last piece I'll mention is I think what's worked well for us is to start simple and grow.
This is particularly true I think with generative AI. Maybe less so on the traditional machine learning side of things, but I think when it comes to generative AI, to really look at it as an ongoing journey, looking at iteration, continuous monitoring and measurement of success, testing it on small groups of users before moving to large-scale is very, very important for a couple of reasons, not least of which is understanding how users actually interact with the technology once it's available to them.
And so I think this concept of iteration and continued growth is going to be very, very important when it comes to the generative AI. I think on the more traditional side like traditional predictive modeling and analytical programs, obviously, can lend themselves to a bit more kind of direct implementation, but on the generative AI front for sure, I think this test and learn approach is going to be very, very important to understand what's working and what's not.
MARC PFEIFFER: Thanks, Paul. From my side, I would say make sure you start with the right use case. Think about what might be again the best cost saver, best risk mitigant, best revenue opportunities as kind of the demand side in there and then understand your capability to address that and the risks you might incur by addressing that.
The second piece, data quality. I mentioned it a few times today, because it's so near and dear to me and having really good high-quality data makes all the difference in the world. Make sure you capture that, make sure you have processes and programs in place to capture that, that your organization, the folks who are entering the data or however you collect that data is done in a consistent matter, it's reliable, etc. and those are going to be good foundations going forward.
Once you've built your AI model on the back end, that implementation is such a critical aspect. So don't ignore that piece. Think about that during the build, during the planning phase really, it's great once you have your signal, your output of the AI model. How are you going to use that? How does that integrate with day to day? Are those team members ready to receive that? In what form will they receive that? How is that going to impact the process? Etc., etc.
So that's such a critical piece for me, and then, lastly, AI really is an ongoing journey. It really requires continuous iteration and monitoring. The models will degrade over time. I think that's normal as your environment changes. Things change around you. That's going to be critical to just make sure, even if nothing has changed within your company, there's a law or something with your competitor that has changed, you need to keep a tab on everything.
SEAN ELLERY: That's great. Thank you both. Awesome discussion. Tons of great points to take away.
Certainly thinking about who should be around the table has been one as we've gone through our journey, having more people around it as well as different perspectives, covering areas like HR, risk, technology, and just some other parts that maybe you hadn't thought about around legal, it's been great to make sure we get the right perspective but challenge our perspective really early on to make sure that we have ongoing success.
Thank you for everyone who took time out of your day to join us.
Soon you'll receive a follow-up e-mail from BMO with a link to a survey for this session and a letter for your CTE credit.
We'll also provide a link for the replay of this webinar which we encourage to share with your team and colleagues.
Hopefully, this webinar starts as a good conversation starter about how AI can benefit your business now and going forward. Have a great rest of your day, everyone, and thank you.
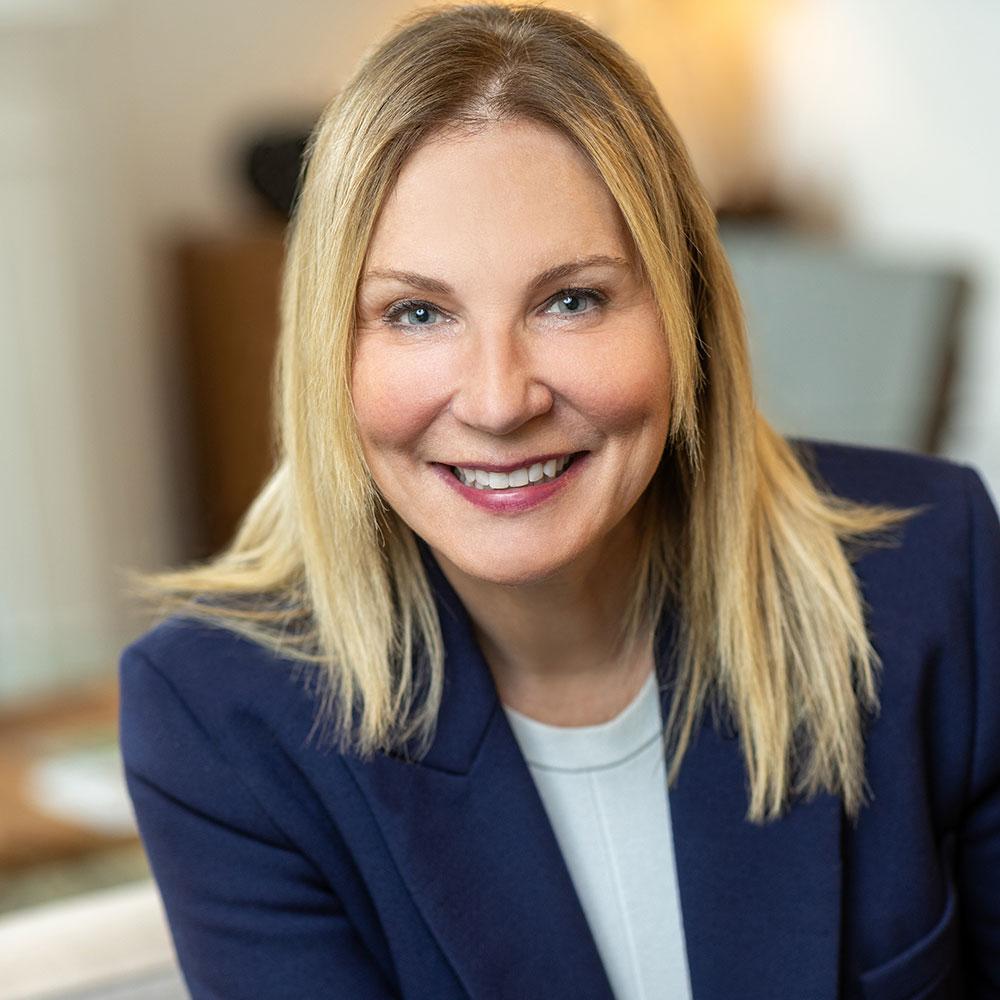
Susan Witteveen
Senior Vice President & Head, Treasury & Payment Solutions
416-643-4549
Susan Witteveen is an accomplished executive within the financial industry across North America, having spent over 20 years in a variety of leadership roles. …(..)
View Full Profile >Contents:
Businesses of all stripes are exploring opportunities to use artificial intelligence (AI) to help solve complex customer problems. Most companies know that AI is important, but they’re not exactly sure how it could make their operations more efficient or how to keep their business safe.
To get beyond the noise, we hosted a webinar with a pair of BMO experts to discuss how companies can harness this emerging technology, including which stakeholders should be involved in the decision to integrate AI into your operations. Our panelists were:
- Paul O'Donovan, Head, Data Analytics and AI Risk Management, BMO
- Marc Pfeiffer, Head, Data Foundations, Innovation and AI, BMO
Sean Ellery, Head of Digital & Innovation Commercial Banking, BMO, moderated the discussion. Following is a podcast and summary of their insights.
Markets Plus is live on all major channels including Apple, and Spotify
.
Start listening to our library of award-winning podcasts.
AI’s business use cases
AI has been around for about 70 years, but advances in computational capabilities and the more recent focus on generative AI tools such as ChatGPT and Google Gemini have made it a part of mainstream conversation. And over the last several years we’ve all been encountering AI in one form or another in our daily lives, whether it’s the auto-correct feature on your mobile phone’s texting app, what-to-watch-next recommendations on streaming TV services, digital personal assistants, or smart home devices. These tools are largely designed for convenience.
From a business perspective, Pfeiffer said AI's potential benefits are largely focused on three areas: cutting costs, mitigating risk, and increasing revenue. To achieve these outcomes, he explained that various industries use AI to provide insights for making better decisions.
It helps you understand the patterns you’re seeing in the data more quickly, with more detail and with more nuance, and then getting those insights from management to customers in terms of spotting trends or making recommendations.
-- Marc Pfeiffer, Head, Data Foundations, Innovation and AI, BMO --
O’Donovan noted that while many companies are leveraging generative AI's automation capabilities to improve efficiency in certain tasks, such as document review, the next step in AI’s evolution will be the transition from a tactical tool to a strategic enabler. “The question that remains is that once these tools are in place and these efficiency gains start to get realized, how do you turn that into hard dollars and cents in terms of an impact to your bottom line,” O’Donovan said.
AI’s limitations
The potential benefits of AI are significant, but so are the possible pitfalls. Pfeiffer pointed out that responsible use starts with a foundation of quality data.
“If you have poor data, if it’s not captured properly, if it’s somehow biased, those are things that are going to lead to inaccurate predictions or faulty decisions,” Pfeiffer said. “One of the things we think a lot about with AI is explainability. You don’t necessarily need to know why the AI decided that a certain Netflix show was a good recommendation. But in other situations, it would be critical to understand why that recommendation or outcome was made. You have to be careful about which AI model you use; some have good explainability, some don’t. It’s something you need to think about, particularly in regulatory or internal compliance situations.”
O’Donovan noted that the risk of biased data is especially important to keep in mind.
These are complex systems that have a lot of black box elements to them. Understanding how your data is moving within the system, how you’re making sure it’s not treating customers or employees in different ways across different protected classes is critical.
-- Paul O'Donovan, Head, Data Analytics and AI Risk Management, BMO --
Upskilling treasury departments
That’s why as more companies adopt AI, some upskilling will be required within corporate treasury departments. Everyone won’t need to become computer scientists, but data literacy, critical thinking and ethics awareness will become essential skills.
As an example, O’Donovan said treasury teams will need the ability to spot where AI functions are not working as expected and know how to relay that information to the development team. “I do see some need for training folks within treasury departments to be able to appropriately interact with the AI system and be able to interpret and flag the output,” he said.
Pfeiffer also cautioned against overreliance on AI, particularly in situations that require nuanced communication, such as customer service.
If you have a chatbot communicating with a client, you need to be able to recognize when an AI isn’t able to handle a question and that it needs to go to a human who has a more nuanced understanding of the business
-- Marc Pfeiffer, Head, Data Foundations, Innovation and AI, BMO --
In its current state, AI functions well when it supports human efforts and work, so the lines of accountability are clear. “In certain cases where you're dealing with sensitive business processes or sensitive information, it’s critical that AI is seen as an enabler in the decision-making process and not the ultimate deciding factor,” O’Donovan said.
Who should be at the table?
As businesses look to leverage AI to support strategic needs, involving key stakeholders early in the process and communicating your goals are crucial to a successful implementation.
Many parts of the organization can be impacted. Ensuring that you have that buy-in early on is going to be a critical factor.
-- Paul O'Donovan, Head, Data Analytics and AI Risk Management, BMO --
To that end, O’Donovan listed the key groups that should be engaged in these discussions:
- Risk management
- Procurement
- Legal
- IT
- Risk and compliance
- Human resources
- Third-party technology providers
Along with getting the right stakeholders involved, cross-collaboration among these groups is also crucial. “What has really worked well is collective engagement,” O’Donovan said. “There’s a lot of overlap between the legal group and the third-party group, the technology group and the risk group. It’s important to have folks in the same room at the same time to make sure that all the different perspectives can be heard.”
Involving all your key stakeholders will help you navigate all the necessary considerations for a successful implementation. That includes making sure you’re using AI to solve for the right use case and that you’re using high-quality, structured data. It also means understanding how AI may impact your operations.
“The AI model is half the challenge,” Pfeiffer said. “The other half is making sure that what you're building will meet the needs of your business. Making sure you understand how it will be used, how the user—whether it's an employee or customer—is going to receive it, and how that's going to change their own daily operations as they interact with the company. Employee training and change management are critical pieces to making sure you understand the post-implementation functionality.”
Ultimately, knowing what you want AI to help you accomplish will drive all the other decisions that your key stakeholders will be involved in. “Companies tend to be less successful when there's not a clear set of criteria for success at the outset,” O’Donovan said. “Being clear on what constitutes a good outcome needs to be clear from the beginning so that all the different partners can be aligned on what the ultimate success looks like.”
What to Read Next.
Four M&A Questions for CFOs to Make Treasury Operations More Efficient
Susan Witteveen | September 05, 2024 | Manage Cash Flow
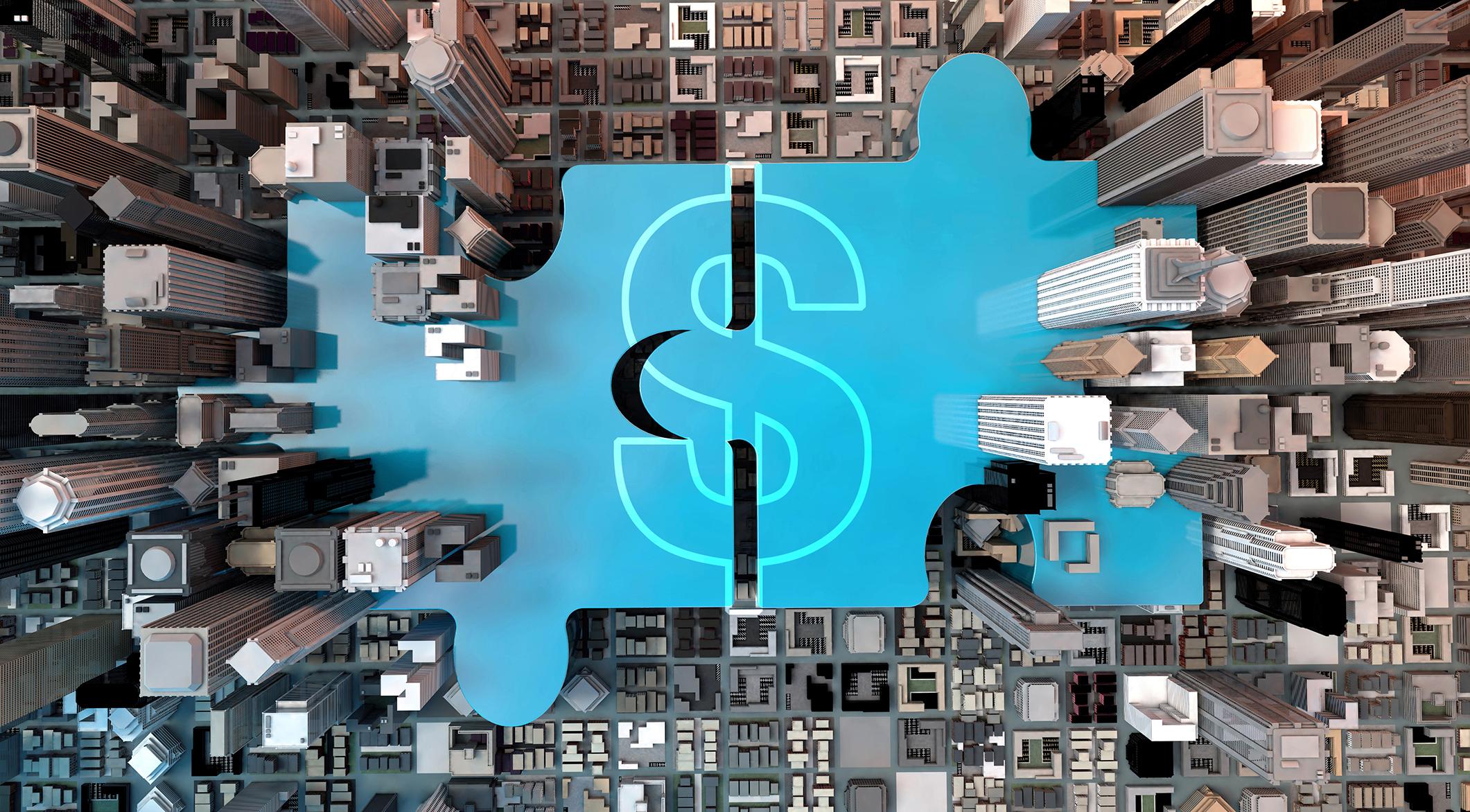
Contents: Leaning on financial partners Four questions to consider Focus on strategy, not products M&A activity is picking up, a…
Continue Reading>Related Insights
Tell us three simple things to
customize your experience
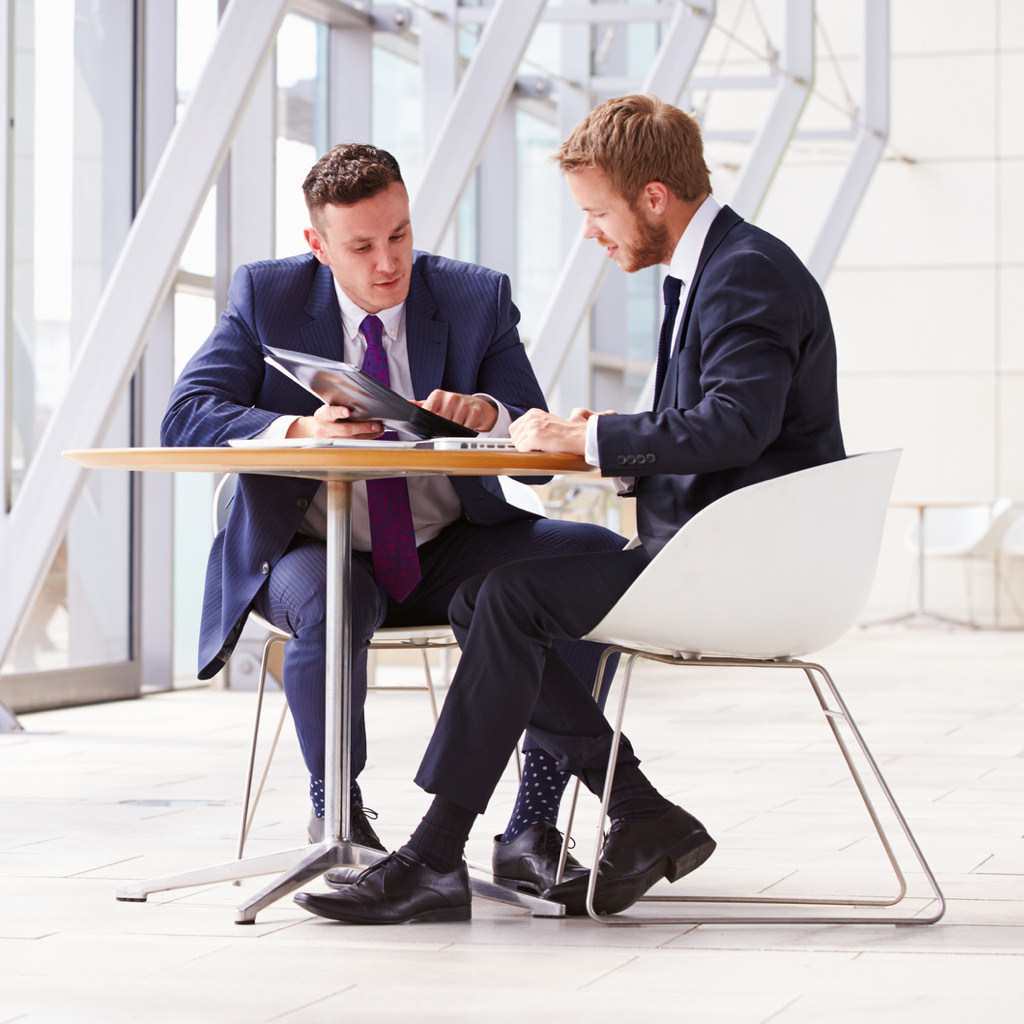
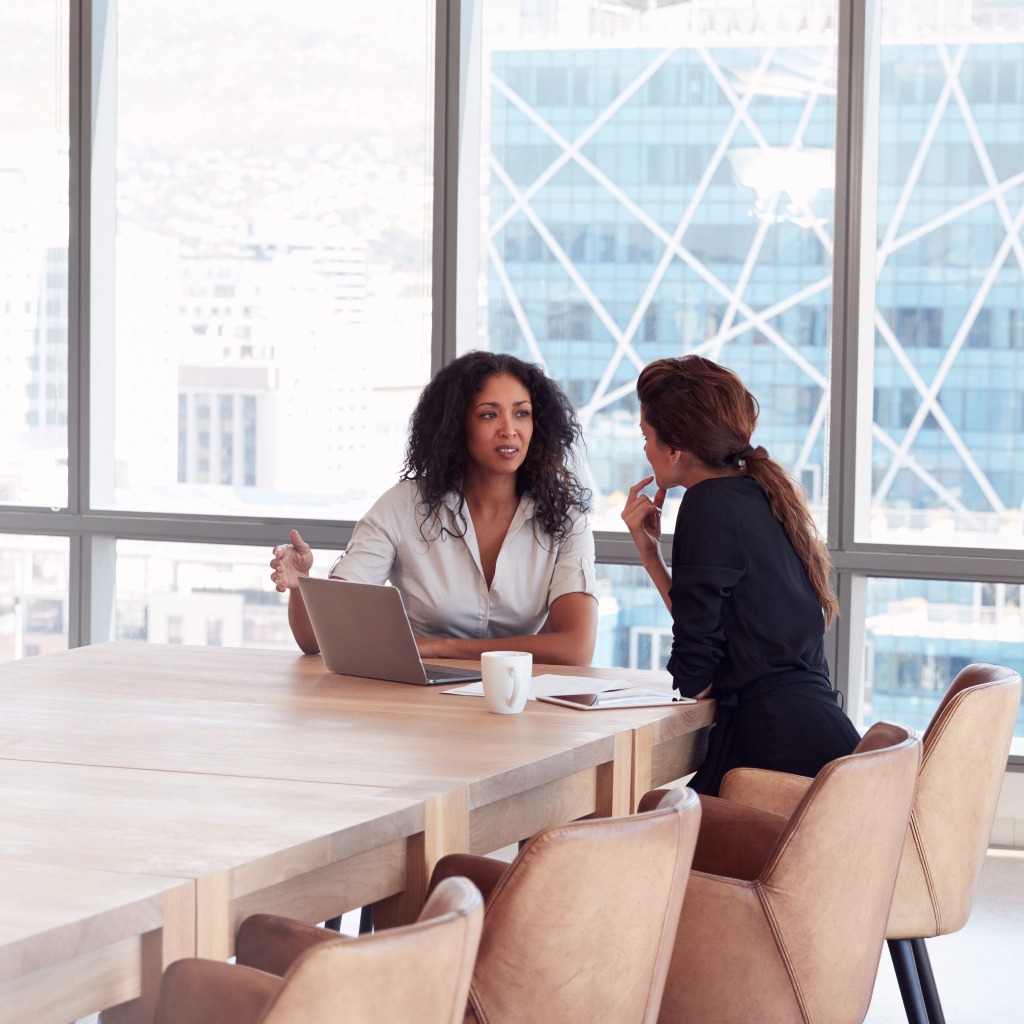
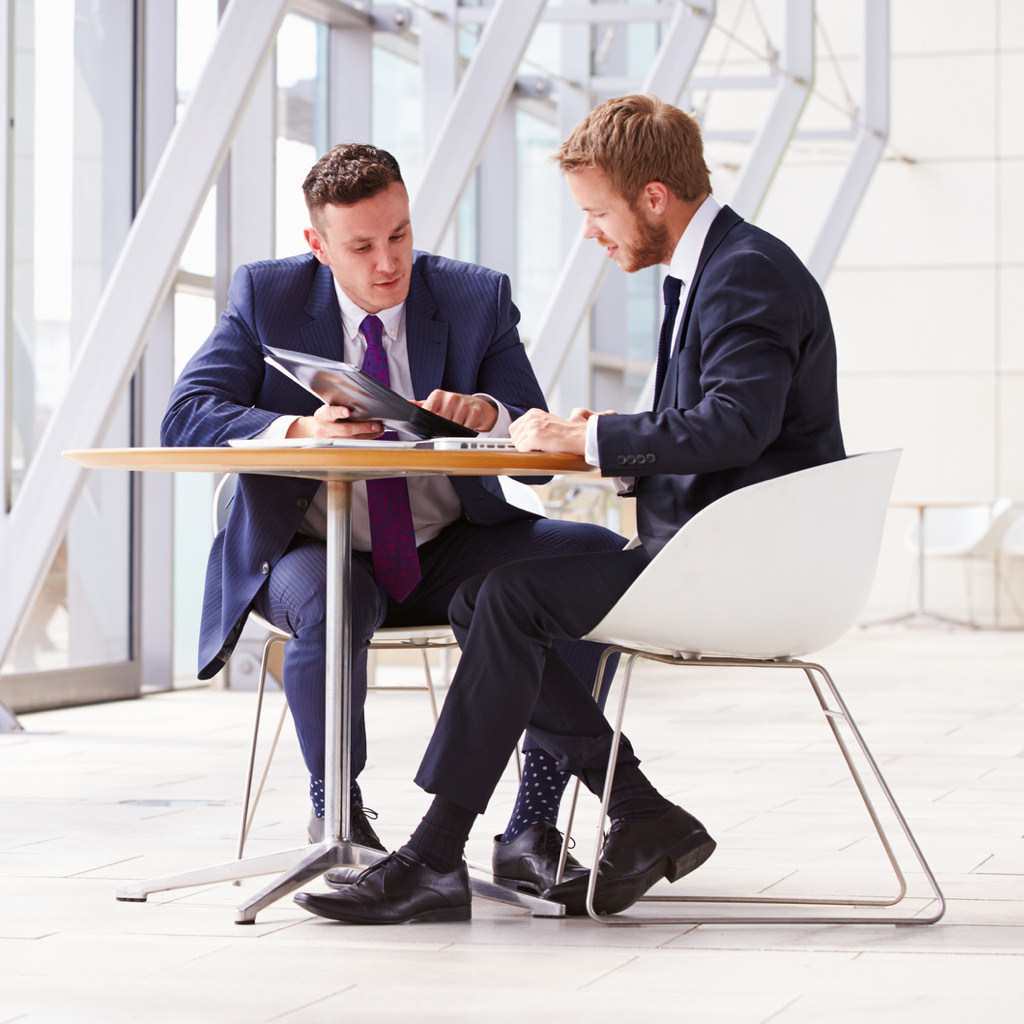
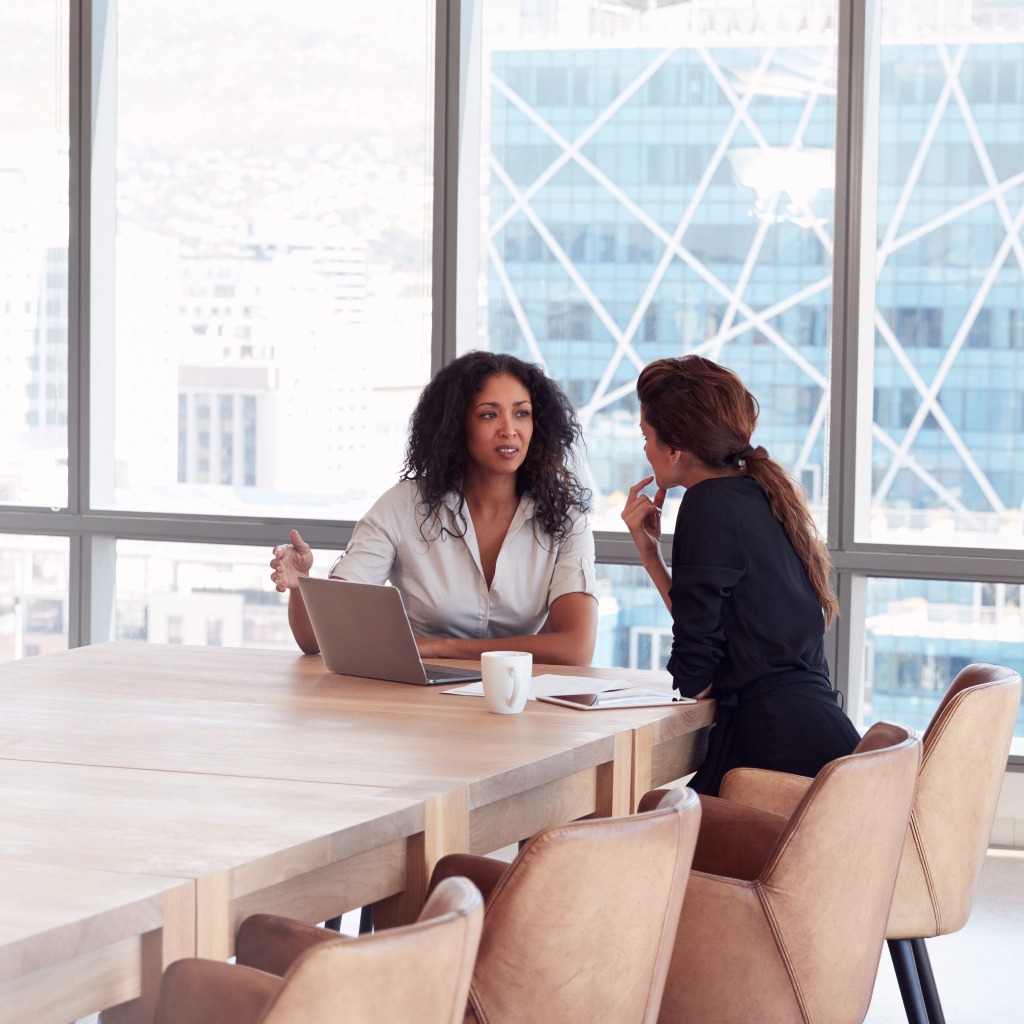
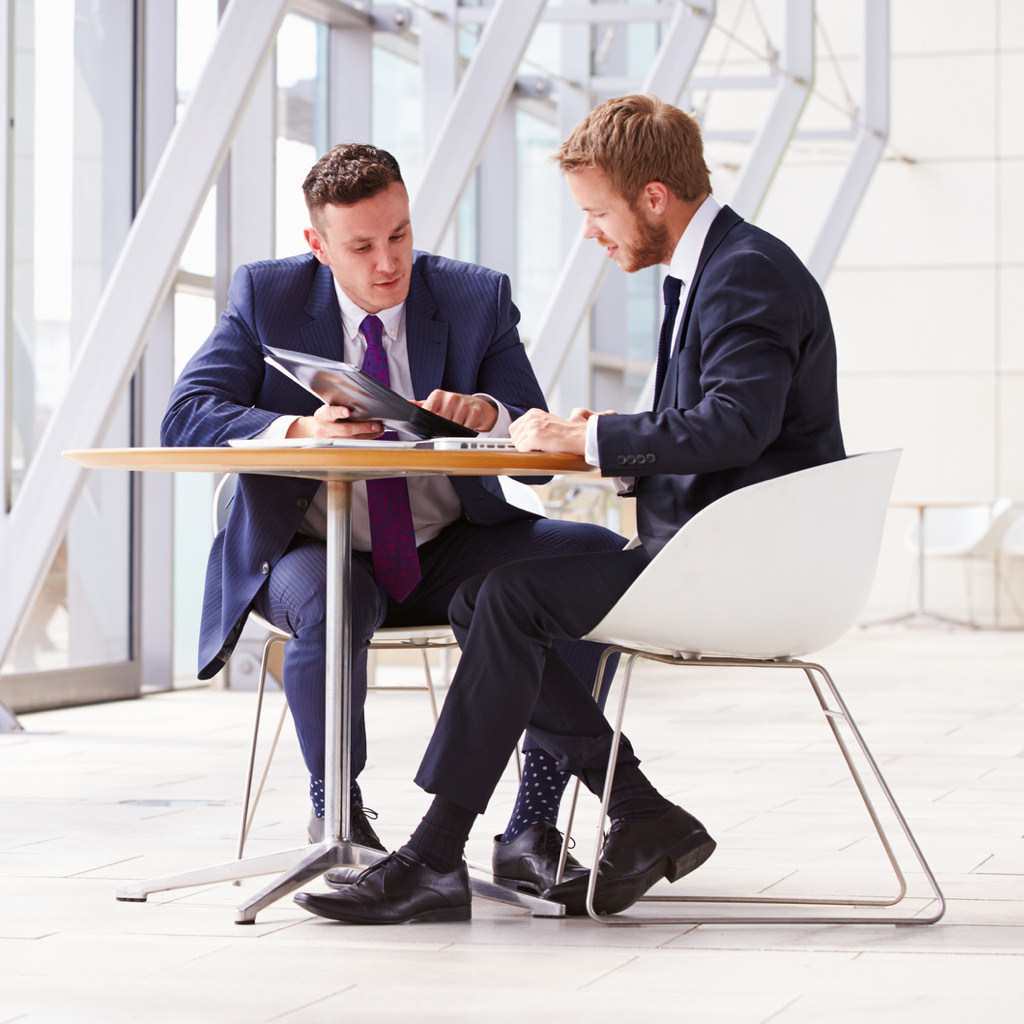
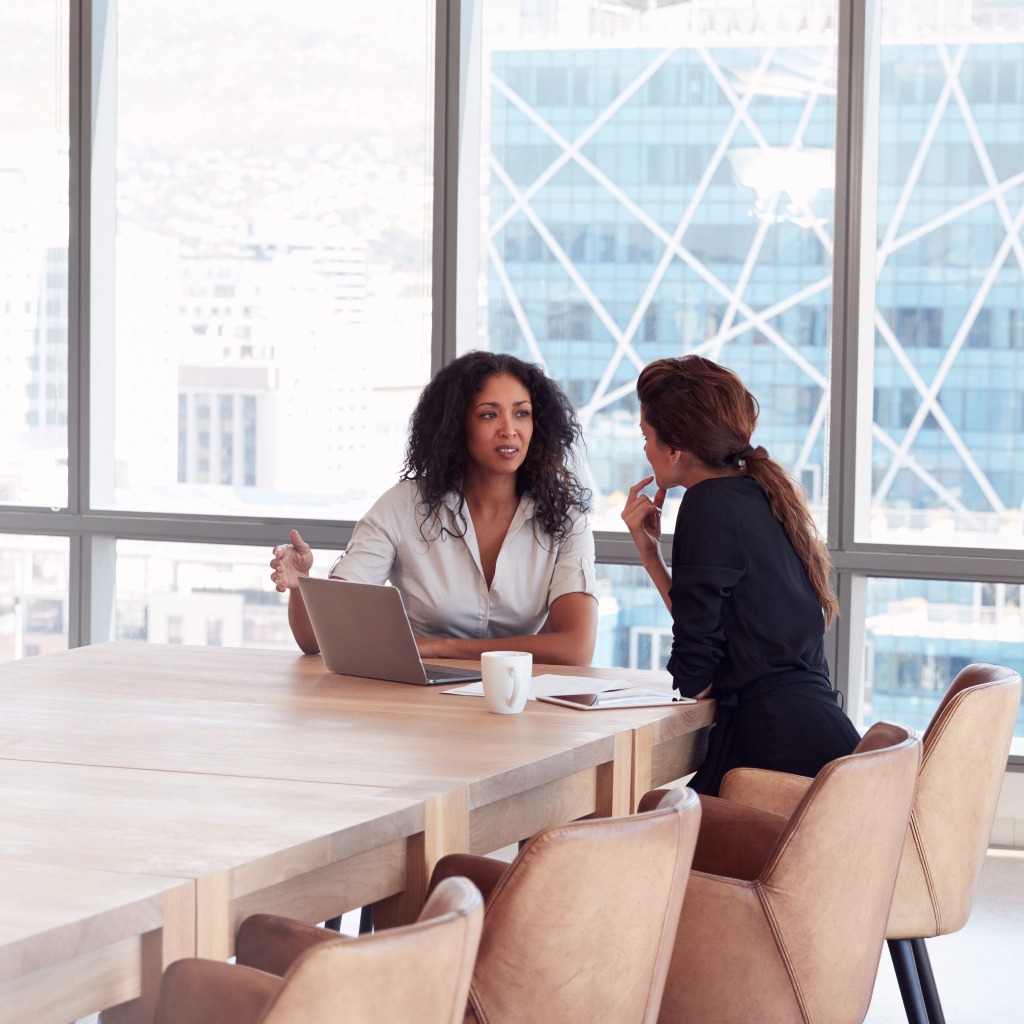
Banking products are subject to approval and are provided in Canada by Bank of Montreal, a CDIC Member.
BMO Commercial Bank is a trade name used in Canada by Bank of Montreal, a CDIC member.
Please note important disclosures for content produced by BMO Capital Markets. BMO Capital Markets Regulatory | BMOCMC Fixed Income Commentary Disclosure | BMOCMC FICC Macro Strategy Commentary Disclosure | Research Disclosure Statements
BMO Capital Markets is a trade name used by BMO Financial Group for the wholesale banking businesses of Bank of Montreal, BMO Bank N.A. (member FDIC), Bank of Montreal Europe p.l.c., and Bank of Montreal (China) Co. Ltd, the institutional broker dealer business of BMO Capital Markets Corp. (Member FINRA and SIPC) and the agency broker dealer business of Clearpool Execution Services, LLC (Member FINRA and SIPC) in the U.S. , and the institutional broker dealer businesses of BMO Nesbitt Burns Inc. (Member Canadian Investment Regulatory Organization and Member Canadian Investor Protection Fund) in Canada and Asia, Bank of Montreal Europe p.l.c. (authorised and regulated by the Central Bank of Ireland) in Europe and BMO Capital Markets Limited (authorised and regulated by the Financial Conduct Authority) in the UK and Australia and carbon credit origination, sustainability advisory services and environmental solutions provided by Bank of Montreal, BMO Radicle Inc., and Carbon Farmers Australia Pty Ltd. (ACN 136 799 221 AFSL 430135) in Australia. "Nesbitt Burns" is a registered trademark of BMO Nesbitt Burns Inc, used under license. "BMO Capital Markets" is a trademark of Bank of Montreal, used under license. "BMO (M-Bar roundel symbol)" is a registered trademark of Bank of Montreal, used under license.
® Registered trademark of Bank of Montreal in the United States, Canada and elsewhere.
™ Trademark of Bank of Montreal in the United States and Canada.
The material contained in articles posted on this website is intended as a general market commentary. The opinions, estimates and projections, if any, contained in these articles are those of the authors and may differ from those of other BMO Commercial Bank employees and affiliates. BMO Commercial Bank endeavors to ensure that the contents have been compiled or derived from sources that it believes to be reliable and which it believes contain information and opinions which are accurate and complete. However, the authors and BMO Commercial Bank take no responsibility for any errors or omissions and do not guarantee their accuracy or completeness. These articles are for informational purposes only.
Bank of Montreal and its affiliates do not provide tax, legal or accounting advice. This material has been prepared for informational purposes only, and is not intended to provide, and should not be relied on for, tax, legal or accounting advice. You should consult your own tax, legal and accounting advisors before engaging in any transaction.
Third party web sites may have privacy and security policies different from BMO. Links to other web sites do not imply the endorsement or approval of such web sites. Please review the privacy and security policies of web sites reached through links from BMO web sites.
Please note important disclosures for content produced by BMO Capital Markets. BMO Capital Markets Regulatory | BMOCMC Fixed Income Commentary Disclosure | BMOCMC FICC Macro Strategy Commentary Disclosure | Research Disclosure Statements