How to Look Beyond the Fear and Hype of AI
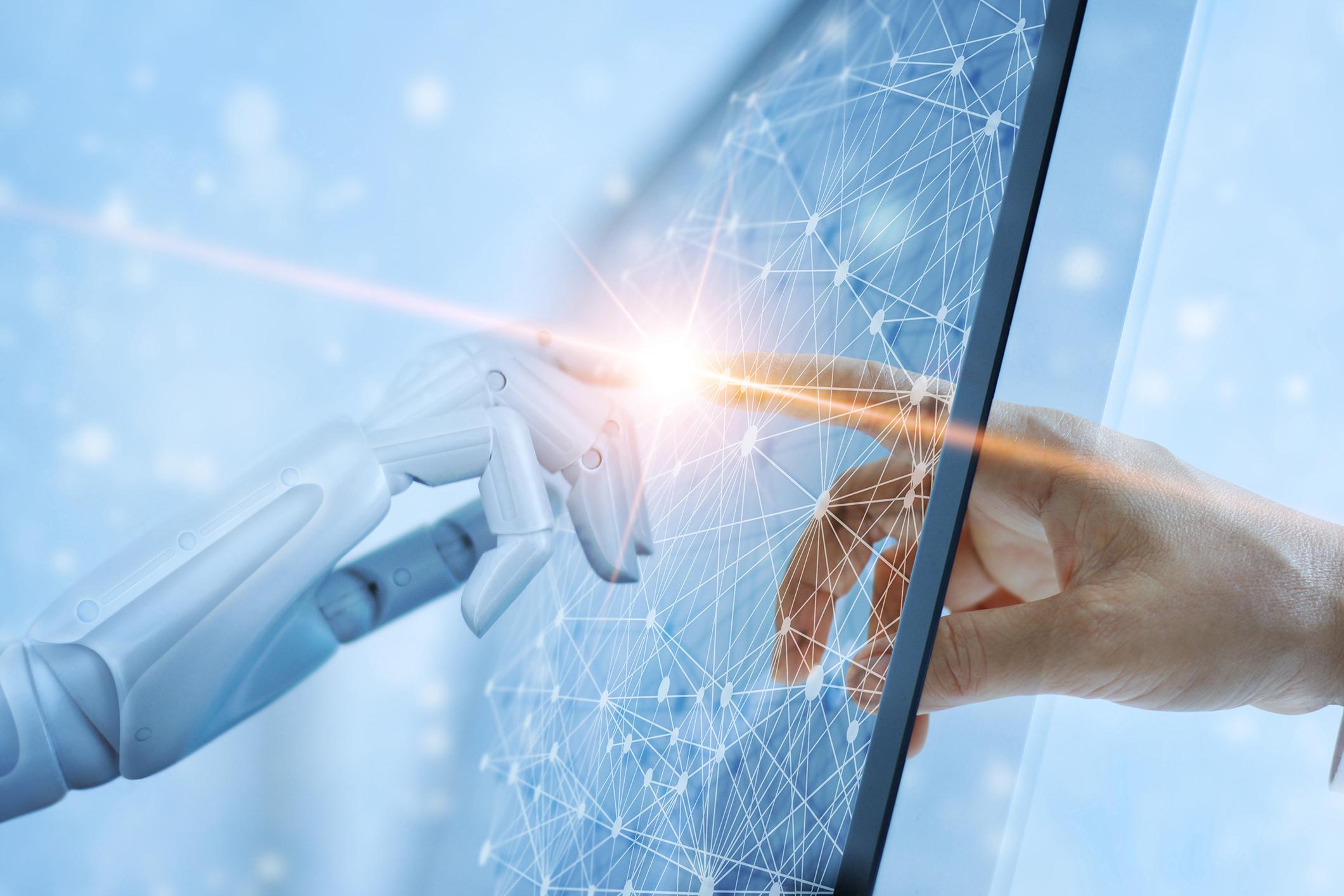
Contents
It’s mind-boggling to think about how quickly artificial intelligence (AI) has come to dominate conversations about the future of business. Until last year, discussions around AI were mostly limited to niche areas of the tech community. Now, generative AI tools built on large language models (LLMs), such as ChatGPT, Microsoft Copilot and Google Gemini, are in the headlines daily.
The changes this technology has brought are already impacting business decisions and will continue to do so well into the future. But making your way in this new world will require looking beyond the headlines—both the overhyped promises and the dystopic fears—and considering how the reality of the current AI models could apply to your business. Ultimately, understanding the risks of their capabilities, the resources they require, and their limitations are as important as being ready to capitalize on the opportunities they present.
Real-world use cases
For now, most of the current use cases for generative AI involve experimentation for improving internal productivity, such as sales and marketing teams using ChatGPT to generate high-level marketing content, note summaries, or email drafts. Currently, there’s been significantly less AI adoption on the customer-facing side, largely because of the legal and regulatory risks involved. But even internal usage can provide external-facing benefits.
For example, you can use an AI sales intelligence system to record calls, create transcripts and examine those transcripts to recognize specific themes, such as pricing and product or service recommendations. From there, you could create personalized marketing campaigns and sales strategies.
Improving individual productivity on specific tasks is one thing. Translating that into bottom-line improvements from a revenue-generating perspective is a more difficult proposition. That’s largely because it involves moving beyond the current crop of LLM-based platforms to AI systems powered by internal data designed to perform functions specific to your business.
In this case, companies need to consider whether they have the technological capacity to run AI systems based on their internal data. While computing power has come a long way, it still isn’t where it needs to be for most organizations to adopt AI easily. It requires significant investment in infrastructure and talent, as well as vast amounts of clean, mature data well beyond what most organizations currently have. Unless your business processes are already digitized, for example, it’s quite difficult to create the necessary data sets to drive the functions you need.
In other words, it will take a strong financial and time commitment for companies to fully leverage this type of AI. But there are several startups developing solutions designed to solve for those problems. Once they become widely available, that will help drive mass adoption of AI systems within organizations.
Be patient, but not complacent
There are many areas where a business would want to capitalize on the first-mover advantage. But because AI is evolving so rapidly, for many companies there may not be much of an advantage to being an early adopter. For most, it’s probably prudent to let the technology mature, giving you time to understand precisely how to govern and manage the technology when you do adopt it.
But that doesn’t mean you shouldn’t experiment with AI in the meantime. Organizations that don’t start learning about it now won’t be able to make up for that time in the next few years. And it doesn’t necessarily require making a large financial investment.
The workforce impact
Business leaders aren’t the only ones who'll have to adapt to AI. Employees will need to consider how AI could impact their current roles and future job prospects.
There’s no immediate panic warranted about potential widespread job losses, but it does mean employees should start getting familiar with AI tools. Experimentation could include having employees use ChatGPT to assist with tasks outside their main job functions, such as using it to design a workshop agenda for an internal team onsite. It’s clear that many jobs will require regular training and, in some cases, re-education as the technology evolves.
Historically, however, the U.S. economy has reacted positively to previous technological revolutions. While it forces workers to adapt, it has also consistently resulted in the creation of new jobs, cost savings, and new revenue opportunities.
One thing is certain: business adoption of AI should not be driven by chasing the newest shiny technology, or using AI for the sake of saying you use AI. But if your path to AI is grounded in understanding how you can use it to create internal efficiencies or solve customer problems, you’ll be putting your organization in a position to capitalize on real-world solutions that can boost revenue as well as efficiency.